Work with momentum—go farther with velocity
At Velocity Global, we’re building a dream team made up of the world’s best talent. We’re looking for people like you.
Working as part of our global company alongside people around the world, you’ll get the opportunity and support to take your career anywhere. Sharpen your existing skills and gain new ones. Access standout perks and resources so you can work in a way that works for you. Never stop expanding your orbit. Together, we can keep learning, growing, and transforming the way the world works.
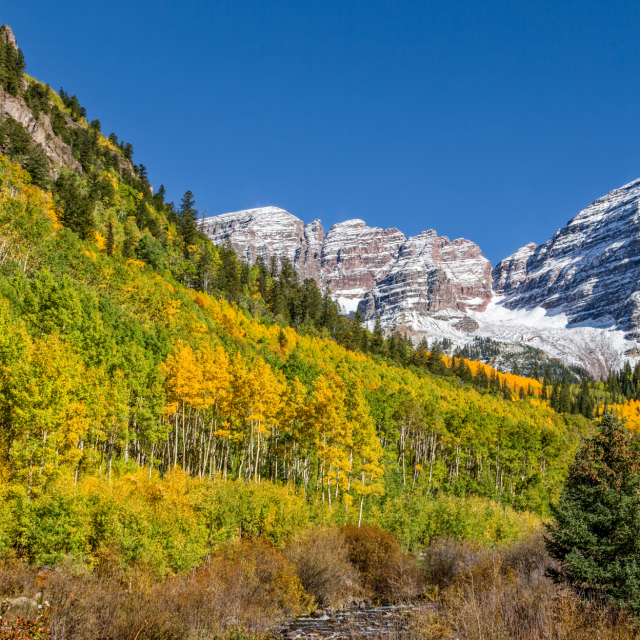
We prioritize a diverse and inclusive environment
Velocity Global cultivates a culture that embraces diversity, equity, inclusion, and belonging (DEIB). Through our company values and employee resource groups (ERGs), we foster a supportive working environment where individuals feel valued and respected.
Pride ERG
Our PRIDE ERG supports the global network of LGBTQIA+ employees and provides them with a safe space in which they can show up to work in their own authentic ways without fear of repercussions.
WIN ERG
The push for equality within gender is still alive, and our WIN (Women’s Initiative Network) ERG serves as a beacon of empowerment, solidarity, and support.
NeuroUnity
This group provides support and advocacy to neurodiverse employees by addressing the challenges and biases they face, such as sensory sensitivities, communication differences, and executive functioning differences.
S.O.U.L
S.O.U.L (Strengthening our Unity and Leadership) is a supportive network dedicated to fostering belonging, unity, and growth for Black employees. Through community engagement, S.O.U.L amplifies voices, promotes diversity, and celebrates Black culture to drive meaningful change.
Get a global perspective with our resources
Open Positions
About Velocity Global
At Velocity Global, our values represent who we are and the company we want to be. We harness the power of our values to bring our unique talents together in pursuit of our common goals. In partnership with our customers and ourselves, we are better together, and together, we win.
We are dedicated to fostering diversity and inclusion across our organization, embracing the rich tapestry of cultures, backgrounds, and perspectives that our global team brings together in offices around the world. Velocity Global is an Equal Opportunity Employer committed to empowering individuals from all walks of life to achieve their professional goals with us, regardless of race, religion, gender, gender identity, pregnancy, disability, sexual orientation, age, national origin, citizenship status, or genetic information. We actively seek and encourage applications from diverse candidates, including those with disabilities, and offer accommodations throughout the selection process upon request.